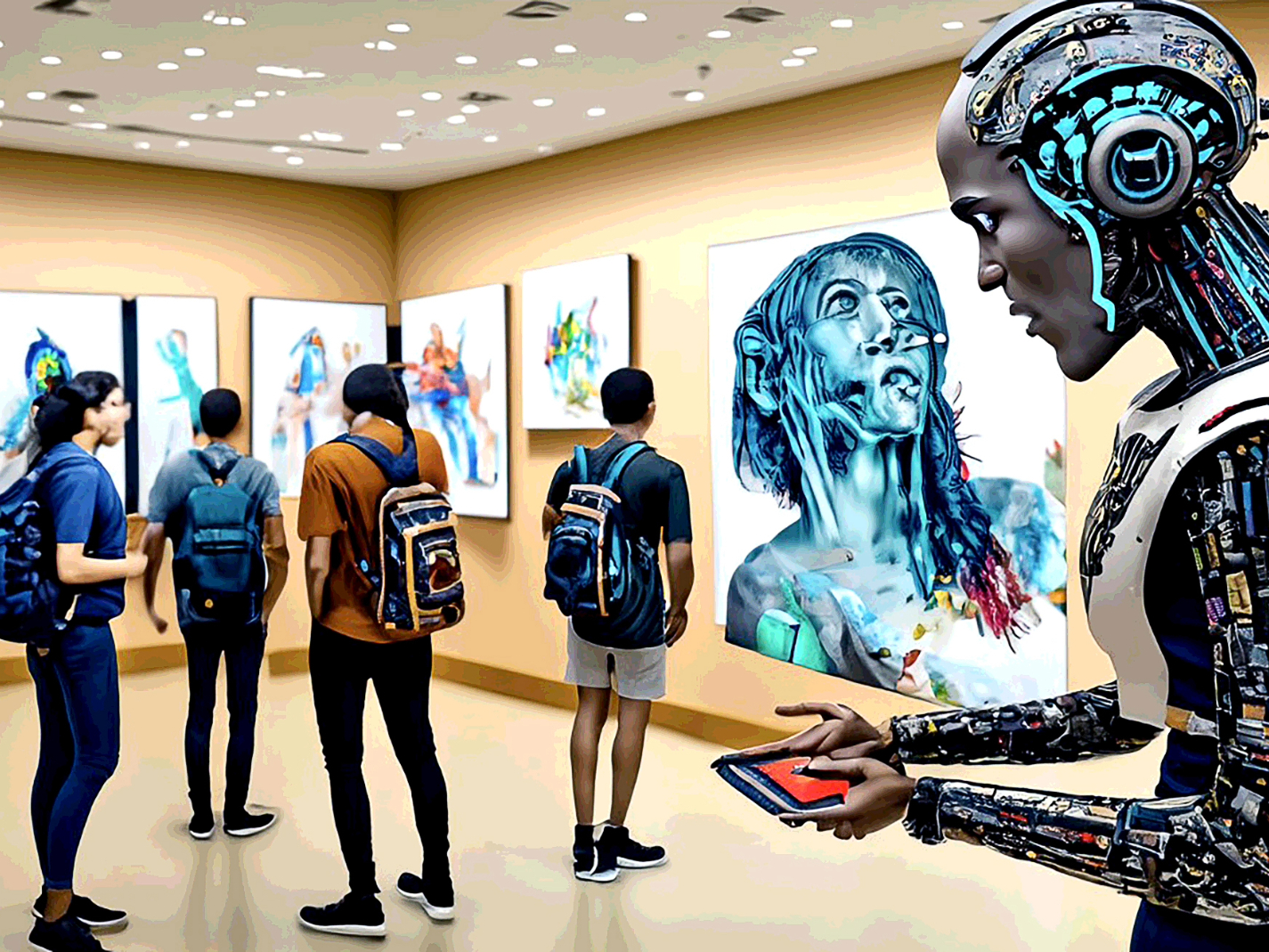
This marks the beginning of a two-part series by digital artist Joe Nalven who explores the integration of AI in campus art galleries as an approach to supersede academic silos.
Just a few years ago, maybe just one year ago, this article would have been considered unrealistic or fantasy—certainly not worthy of administrative attention nor a funding opportunity.
Now, we are witnessing an AI deluge in and out of academia—no discipline will be untouched. The meaning of a college education requires a new template, and we don’t really know what that template is or should be.
I have written several articles with chatbot interlocutors, extending my digital artistry with generative artificial intelligence models. Like most readers, I have consumed articles on the promise and dangers of AI—the way they operate, their emergent capabilities, the way they can be exploited by bad actors, the commercial impetus to release ever more advanced AI models, the absence of a serious regulatory framework that does not crush the innovative spirit, military applications, and much more.
What is clear is that AI has and will continue in ever more penetrating ways into how we live our lives. In academia, that includes how classes are taught, how students learn, how research is done and reported, and how administrators will reconfigure the educational institution.
AI can be a method to reconfigure university education—not in its totality, but as a way to integrate and reintegrate learning across disciplinary silos.
Looking back to the medieval university, seven liberal arts were required for a degree—arithmetic, geometry, astronomy, music theory, grammar, logic, and rhetoric. Today’s university has dozens of disciplines, stylized jargon, and research methods tailored to a plethora of objectives. These disciplines are managed more often than not as separate fiefdoms or silos. Understandably, such educational silos can have advantages in framing objectives and process, but they can shield the larger educational enterprise from cross, multi, trans, and inter-disciplinary practices—not to mention how education maps onto reality outside the university.
AI can supersede these silos.
All communication within each and every discipline—whether mathematical symbols, human language, research reports, publications, lectures, designs, plans, robotics, image generation, speech recognition, computer vision, and on and on—is reducible to code. Machine language, now structured as Large Language Models (LLM), can talk across these ostensibly separate fields of inquiry.
This is where unanticipated AI capabilities emerge.
We separate data into sound, vision, touch, smell, and taste because that is how humans channel perception. AI models are not bounded in this way, however. So, with all communication and all information potentially being part of a LLM, the same could be true of university education—those walls come down, and the silos are deconstructed.
I consulted Bard, an AI chatbot, asking it, “Would AI LLMs undermine academic silos since LLMs code all information that can cross disciplinary boundaries?” This was its response:
Yes, AI LLMs have the potential to undermine academic silos in several ways.
-
- LLMs can access and process information from a wide range of sources. This includes not only academic journals and books, but also news articles, websites, social media, and other forms of unstructured data. This gives LLMs a much broader perspective than any human expert could possibly have.
-
- LLMs can identify patterns and relationships in data that humans would miss. This is because LLMs can process information much faster and more efficiently than humans can. As a result, LLMs can make connections between different disciplines that humans might not even see.
- LLMs can generate creative solutions to problems. This is because LLMs are not limited by the same constraints as human experts. LLMs can explore new ideas and possibilities without being bound by traditional disciplinary boundaries.
As a result of these capabilities, AI LLMs have the potential to revolutionize the way we do research and scholarship. They can help us to break down barriers between different disciplines and to make new discoveries that would not have been possible otherwise.
One way to avoid silos is a university without walls—a university that is shaped by policy can work cross-disciplinary interests. The policy objective would also sit comfortably with AI and LLM engines. A policy, such as climate change, would optimize congruent research and application, deterring the insularity found in academic silos.
A policy framework is not necessary to have a university without walls. More broadly, we should be speaking of an education without walls. It can be re-integrated around AI and LLM engines without an additional policy framework. The difference is similar to basic versus applied research. In both contexts, academic silos will be pressured by AI’s universalizing and networking data context to become permeable.
A methodology to visualize AI’s impact on academic silos
The premise of this essay and the one to come is that AI will pressure academic silos to have greater permeability but could be challenged as hypothetical and without sufficient grounding in how academic silos function. Another challenge would be that faculty and administration would raise various defenses as to why their silos are sacrosanct. Putting those likely challenges aside for the moment, it would be interesting to lay out a methodology of how data within these silos might be re-integrated with an AI framework. The elaboration of this proposed methodology could illustrate how disciplines would retain their usefulness in this permeable educational framework.
Wearing an artist’s hat, I propose a campus art gallery as a framework to discuss how AI can re-integrate several university disciplines—art practice, art history, semantics, cognitive science, computer programming, data training for LLMs, bias, law, economics, epistemology, and more. A campus art gallery can reintegrate education with an LLM model that is part curator of existing data and part generative tool that can create new data combinations. Other places in the university can illustrate additional examples of re-integrating disciplinary silos.
In this hypothetical art exhibit, the LLM will co-curate the exhibit with a human.
Donors and political correctness may influence human gallery directors, but in this hypothetical we are imagining a human art gallery director who has been liberated from these constraints. LLMs have limits as well, such as being sanitized with safeguards like no violence, no sexual imagery, privacy, and security. LLMs have other limitations. They are not completely neutered of human bias. There are concerns about the data sets on which they have been trained, as well as faulty assumptions we have about machine learning. So, appointing an LLM as a gallery co-curator does not resolve the real-world limitations of the human juror or curator of art.
A hypothetical art exhibit: Words and Perception: Thesis, Antithesis & Synthesis:
Imagine University X Art Gallery. Students from STEM and liberal arts are invited to visit each of three rooms. The first room explores the arbitrary connection between the words we use and the objects that are described with these words. It shows how an identical object can be perceived differently depending on the words used.
The second room continues the discussion of how we know the world by the words we use to describe it. However, this room takes a different point of departure—the LLM’s text prompt. Individuals describe a thing, a relationship, a process, a style of presentation and the like. In turn, the LLM generates a representation arising from those words based on its database of images, how they have been tagged with metadata (training), and the layers and loops its algorithms use to generate and discriminate among presumed corresponding images— generative artificial intelligence (GAI).
Curiously, if the same words from the first room are used as text-prompts in the second room, the representations deviate, often wildly, from the object in the first room.
The third room in this exhibit is different.
It is based on an existing discipline framed by the materials, techniques, and subjects in making art and how these have changed over time and in different cultures. In this example, the authority figure is the subject matter of each of the artists across five centuries. This room allows the instructor or curator to incorporate art history into the more general question of human representation. Instead of analyzing human representation of word to real-world object—or the way GAI represents objects based on words—this third point of departure turns to the actual human creative development across centuries, namely, the disciplinary knowledge accumulated in art history and related disciplines.
True, the discipline of art history can continue as an academic silo, as the other two rooms are not really needed to explain instances of art history.
However, students and those who use LLMs to generate imagery will wonder about the limits past centuries have imposed and continue to impose on creative efforts. They will ask how human artists can explore unexpected ways in which art-making occurs compared with the speed offered by LLMs.
Speed, unexpected results, and multiple surprises are what we encounter with LLMs. It is a very different kind of engine than what the traditional art history model offers. Therein lies the opportunity to juxtapose history and disciplinary knowledge with what this technology offers.
In this way, the three rooms form a three-legged stool, three points of departure situated in a specific content area. It is not that such cross-disciplinary conversations and studies have not already occurred, nor continue to function without LLMs, but the canvas of un-siloed conversation has significantly broadened.
In the article to follow, we will visit each of these three gallery rooms and test the boundaries of permeability.
Editor’s Note: The visual content accompanying this article, including the cover photo, has been exclusively crafted by the author utilizing Photoshop Generative Fill, DALL·E 3, and Deep Dream Generator that transform text into images.
Courts are having a real problem with AI because it is citing cases that do not exist — fabricating facts. Absent a reason to believe otherwise, one has to assume that it is doing likewise in all other applications, and hence something that one does not want in academia.